The AI Enthusiasm-Application Gap—Lessons from eDiscovery
The Bencher | July/August 2024
By Joseph C. Leonard, Esquire
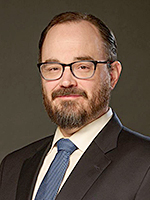
Artificial intelligence (AI) dominates both the popular and professional landscapes, exemplified by the release and subsequent downloads of the generative AI program ChatGPT, resulting in over 100 million users.
Although AI in some form has been used in the legal industry for many years, enthusiasm for practical application of AI models broke through in 2023. AI purports to revolutionize legal work, but expectations should be tempered while education should be prioritized, particularly awareness of pitfalls and costs.
Generative AI
“Generative AI” captured the public’s imagination based on its ability to create clear and polished responses to any question put to it. Generative AI uses large language models, or LLMs, training on the immense volume of data scraped from the internet. These programs feel like an amalgam of oracle and magic lamp, answering questions and conjuring content at the click of a button.
However, some of that luster has worn off in the wake of high-profile “hallucinations,” in which it became clear that the program was not actually considering questions and identifying answers, but rather sketching out a likely response that largely aligned with its training data, using fiction to fill in any gaps in its answer. Frequently, those answers were factually false, as was the case in Mata v. Avianca, Park v. Kim, and the roll-out of New York City’s landlord tenant chatbot.
Historically, the legal profession has been slow to embrace new technologies. However, eDiscovery service provider Lighthouse’s February 2024 “State of AI in eDiscovery” illustrates the legal industry’s high level of interest and appetite for the adoption and integration of AI systems into its workflows, while also highlighting the need for development of company policies and education around the technology. AI represents a great opportunity for the field, but the associated risks must also be clearly understood.
eDiscovery can illuminate potential drawbacks and provide solutions, as the practice has employed predictive AI coding and technology-assisted review for decades. Existing protocols for validation of output result from years of analysis and thought leadership. The key to AI application in eDiscovery is that the process be replicable and defensible.
Predictive AI
“Predictive AI,” such as technology-assisted review (TAR), allows a document review platform to analyze data and promote likely responsive material for review and potential production based on initial “seed” data. On scale, it results in an enormous return on investment, with accuracy higher than human reviewers and likely non-responsive material pushed to the end of the review, where, ultimately, it may not require review at all. The process is replicable because it is validated by statistical analysis measuring both precision (how many documents coded relevant are confirmed relevant) and recall (how many relevant documents are missing from the final set.) However, both sides must have a clear understanding of the process.
Established and transparent validation ensures the project output falls within expected parameters, and both the method and the results are discussed with opposing counsel to ensure everyone is working from a place of full information. Human validation of results is paramount to the successful implementation of AI because without it, it’s impossible to argue that the process is defensible.
At this moment, generative AI recalls early TAR implementation in that most parties are not on equal footing in terms of understanding the tool. Generally, algorithms are closed, and training data are obscured. It’s extremely difficult to explain the process to a lay person, and many experts will admit that they cannot describe exactly what is happening within the LLM. One asks a question of the model and gets a seemingly reasonable answer with the appearance of validity and success, and so may too quickly be deemed as such. In fact, these models return varying responses to the same prompt, calling the replicability of the process into question.
Development in AI implementation over the past year is rapid and exciting, but there is still much work needed before full-scale adoption is practical. Development of firm policies and attorney education regarding AI tools is paramount. Those attorneys with experience in validating AI output should share their expertise with colleagues not familiar with the subject. In turn, those who do not have such experience should reach out to those in the legal technology community to benefit from the knowledge gained in that practice.
Joseph C. Leonard, Esquire, is an associate with Morris James LLP in Wilmington, Delaware, where he leads the firm’s eDiscovery practice and advises clients in electronic discovery and information governance best practices. He is a member of the Richard S. Rodney and the Richard K. Herrmann Technology American Inns of Court.
An expanded version of this article originally appeared in the March 2024 issue of The Journal of the Delaware State Bar Association, a publication of the Delaware State Bar Association. © Copyright Delaware State Bar Association 2024. All rights reserved. Reprinted with permission.